Statistics & Data Science Colloquia are generally held on Monday afternoons. Below is a list of current and upcoming colloquia along with a short history of past events.
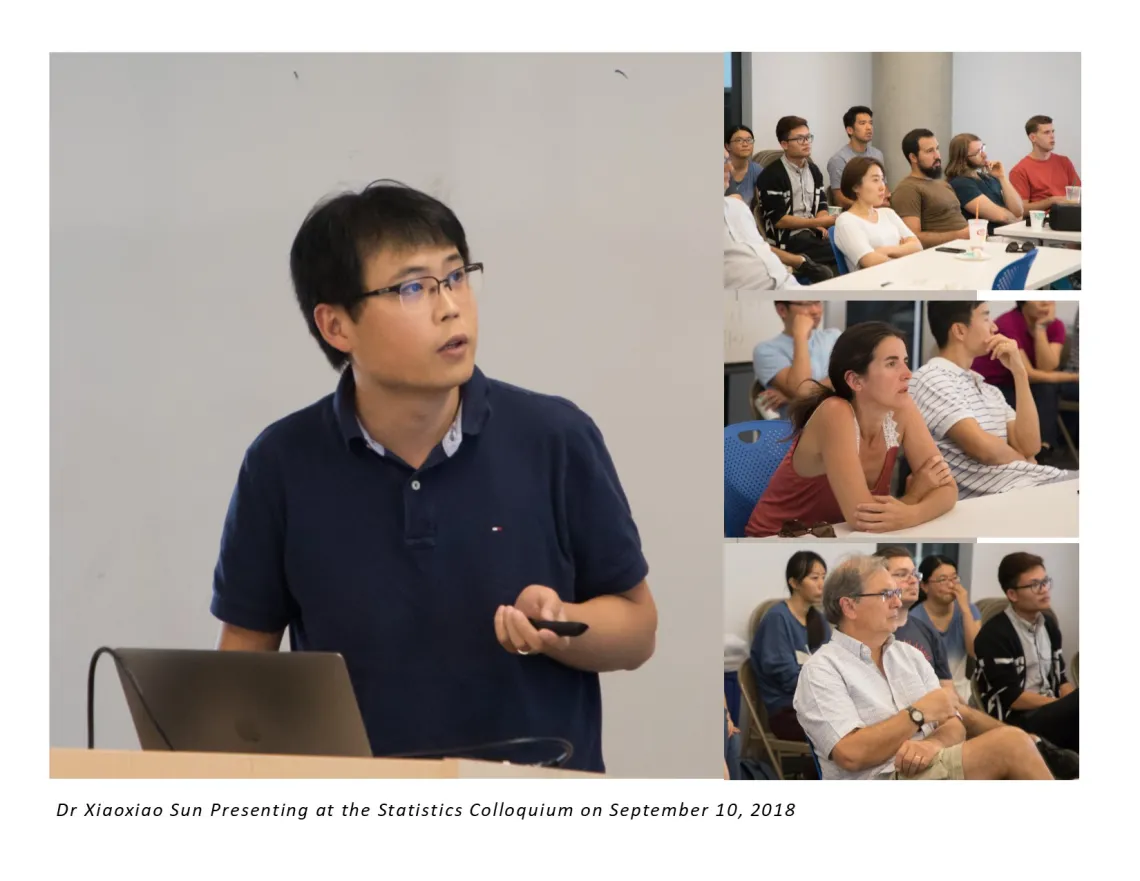
Spring 2025
- February 3, 2025, Speaker Beth Tellman, UArizona School of Geography, Development, and Environment, Title and Abstract
- February 24, 2025, Speaker Jing Lei, Carnegie Mellon University, Department of Statistics and Data Science, Title and Abstract
- March 3, 2025, Speaker Yichao Wu, University of Illinois, Chicago, Department of Math, Science, and Computer Science, Title and Abstract
- April 7, 2025, Speaker Travis Wheeler, UArizona College of Pharmacy, Title and Abstract
Fall 2024
- September 16, 2024, Speaker Andrew Bennett, UArizona Dept of Hydrology and Atmospheric Science, Title and Abstract
- October 28, 2024, Speaker Yong Ge, UArizona Dept of Management Information Systems, Title and Abstract
- November 4, 2024, Speaker Yang Feng, NYU School of Global Public Health, Title and Abstract
- December 2, 2024, Speaker Samy Missoum, UArizona Dept of Aerospace and Mechanical Engineering, Title and Abstract
Spring 2024
- March 11, 2024, Speaker Mingyu Liang, UArizona Dept of Physiology, Title and Abstract
- April 1, 2024, Speaker Dan Cheng, ASU, Title and Abstract
- April 15, 2024, Speaker Mengyang Gu, UC Santa Barbara, Title and Abstract
- April 29, 2024, Speaker Liliana Salvador, UArizona, Title and Abstract
Fall 2023
- September 11, 2023, Speaker Jinchi Lv, USC Marshall School of Business, Title and Abstract
- October 16, 2023, Speaker Wen Zhou, Colorado State University
Spring 2023
- February 20, 2023, Speaker Zhengyuan Zhu, Iowa State University, Title and Abstract
- March 13, 2023, Speaker Afrooz Jalilzadeh, UArizona Systems and Industrial Engineering, Title and Abstract
Fall 2022
- September 12, 2022, Speaker Lifeng Lin UArizona Epidemiology and Biostatistics, Title and Abstract
- October 3, 2022, Speaker James Powell UArizona Economics, Title and Abstract
- November 7, 2022, Speaker Yiwen Liu Uarizona Epidemiology and Biostatistics, Title and Abstract
- December 5, 2022, Speaker Gongjun Xu University of Michigan Department of Statistics, Title and Abstract
Spring 2022
- January 10, 2022, Speaker Henry Zhang, 2:30pm via Zoom. Zoom link, title and abstract.
- January 31, 2022, Noon. Speaker Xiaowu Dai from Berkeley. Location, Title and abstract.
- February 21, 2022, 2:30 Speaker Ning Hao from University of Arizona. Title: Introduction to LaTex and R Markdown, Recording of Event, Statistics Professional Development Series
- February 28, 2022, Speaker Chris Reidy, "Basics of High Performance Computing (HPC)"
- March 14, 2022, Speaker Lupita Estrella, "Grad Path and Program Requirements", Recording of Event
- March 21, 2022, Speaker James Lu, Professional Development Series, "Prepare for Your Statistician/Biostatistician Career: Resume and Job Interview". Recording of Event
- April 4, 2022, Speaker Hongxu Ding, 2:30 hybrid Zoom and PAS 522, Title and Abstract, Recording of Event
- April 18, 2022, Professional Development Seminar: Interpersonal Skills: Oral and written communications. Speaker Laura Miller, UArizona Math Faculty. Recording of Seminar
- April 26, 2022, Professional Development Seminar: Creating an Academic Personal Webpage, Speaker, James Smith, UArizona PhD Student, Recording of Event
- May 2, 2022, Speaker Toby Hocking, 2:30
Fall 2021
- September 27, 2021, Faculty Research Blitz. Recording of event.
- October 18, 2021, 2:30pm - Speaker - Shujie Ma, UC Riverside Recording of event.
Title: Causal inference via artificial neural networks: from prediction to causation
Abstract: Recent technological advances have created numerous large-scale datasets in observational studies, which provide unprecedented opportunities for evaluating the effectiveness of various treatments. Meanwhile, the complex nature of large-scale observational data post great challenges to the existing conventional methods for causality analysis. In this talk, I will introduce a new unified approach that we have proposed for efficiently estimating and inferring causal effects using artificial neural networks. We develop a generalized optimization estimation through moment constraints with the nuisance functions approximated by artificial neural networks. This general optimization framework includes the average, quantile and asymmetric least squares treatment effects as special cases. The proposed methods take full advantage of the large sample size of large-scale data and provide effective protection against mis-specification bias while achieving dimensionality reduction. We also show that the resulting treatment effect estimators are supported by reliable statistical properties that are important for conducting causal inference.
Zoom Meeting ID: 81376809383
- November 1, 2021, 2:30 - 3:30, Speaker - Xin Henry Zhang, Recording of Event
Title Tensor modeling in categorical data analysis and conditional association studies.
Abstract In this talk, we offer new tensor perspectives for two classical multivariate analysis problems. First, we consider the regression of multiple categorical response variables on a high-dimensional predictor. A $M$-th order probability tensor can efficiently represent the joint probability mass function of the $M$ categorical responses. We propose a new latent variable model based on the connection between the conditional independence of the responses and the rank of their conditional probability tensor. We develop a regularized expectation-maximization algorithm to fit this model and apply our method to modeling the functional classes of genes. Second, we consider the three-way associations of how two sets of variables associate and interact, given another set of variables. We establish a population dimension reduction model, transform the problem to sparse Tucker tensor decomposition, and develop a higher-order singular value decomposition estimation algorithm. We demonstrate the efficacy of the method through a multimodal neuroimaging application for Alzheimer's disease research.
Fall 2020 Colloquia will take place on Zoom.
- October 19, 2020, 2-3pm – Speaker - Erfan Yazdandoost Hamedani, SIE, University of Arizona Link to Recording of Colloquium
Title: Primal-dual Methods for Convex-concave Saddle point Problems
Abstract: Recent advances in technology have led researchers to study problems with more complicated structure such as robust classification, distance metric learning, and kernel matrix learning arising in machine learning. In this work, we consider a convex-concave saddle point problem which includes convex constrained optimization problems as a special case. therefore, there has been a pressing need for more powerful, iterative optimization tools that can handle these complicated structures while employing efficient computations in each iteration. This demand has attracted a vast amount of research focusing on developing primal-dual algorithms due to the versatility of the framework. We proposed a primal-dual algorithm and its block coordinate randomized variant with a new momentum term leading to an optimal convergence rate. Moreover, to facilitate the practical implementation of the algorithm a backtracking technique is also proposed. The significance of this work is mainly due to 1) the simplicity of the proposed method, 2) the new momentum in terms of gradients, and 3) its ability to have larger step-sizes compared to the other related work.
- November 2, 2020, 2-3pm – Speaker - Haiying Wang, Dept of Statistics, Univ. of Connecticut, Recording of Event
Title: Maximum sampled likelihood estimation for informative subsample
Abstract: Subsampling is an effective approach to extract useful information from massive data sets when computing resources are limited. Existing investigations focus on developing better sampling procedures and deriving probabilities with higher estimation efficiency. After a subsample is taken from the full data, most available methods use an inverse probability weighted target function to define the estimator. This type of weighted estimator reduces the contributions of more informative data points, and thus it does not fully utilize information in the selected subsample. This paper focuses on parameter estimation with selected subsample, and proposes to use the maximum sampled likelihood estimator (MSLE) based on the sampled data. We established the asymptotic normality of the MSLE, and prove that its variance covariance matrix reaches the lower bound of asymptotically unbiased estimators. Specifically, the MSLE has a higher estimation efficiency than the weighted estimator. We further discuss the asymptotic results with the L-optimal subsampling probabilities, and illustrate the estimation procedure with generalized linear models. Numerical experiments are provided to evaluate the practical performance of the proposed method.
- November 16, 2:30 - 3:30pm – Speaker - Yoonkyung Lee, Dept of Statistics, Ohio State University
Title: Assessment of Case Influence in Support Vector Machine
Abstract: Support vector machine (SVM) is a very popular technique for classification. A key property of SVM is that its discriminant function depends only on a subset of data points called support vectors. This comes from the representation of the discriminant function as a linear combination of kernel functions associated with individual cases. Despite the direct relation between each case and the corresponding coefficient in the representation, the influence of cases and outliers on the classification rule has not been examined formally. Borrowing ideas from regression diagnostics, we define case influence measures for SVM and study how the classification rule changes as each case is perturbed. To measure case sensitivity, we introduce a weight parameter for each case and reduce the weight from one to zero to link the full data solution to the leave-one-out solution. We develop an efficient algorithm to generate case-weight adjusted solution paths for SVM. The solution paths and the resulting case influence graphs facilitate evaluation of the influence measures and allow us to examine the relation between the coefficients of individual cases in SVM and their influences comprehensively. We present numerical results to illustrate the benefit of this approach.
- December 7, 1-2pm – Speaker - Pang Du, Dept of Statistics, Virginia Tech University
Title: A new change point analysis problem motivated by a liver procurement study
Abstract: Literature on change point analysis mostly requires a sudden change in the data distribution, either in a few parameters or the distribution as a whole. We are interested in the scenario where the variance of data may make a significant jump while the mean changes in a smooth fashion. The motivation is a liver procurement experiment monitoring organ surface temperature. Blindly applying the existing methods to the example can yield erroneous change point estimates since the
smoothly-changing mean violates the sudden-change assumption. We propose a penalized weighted least squares approach with an iterative estimation procedure that integrates variance change point detection and smooth mean function estimation. The procedure starts with a consistent initial mean estimate ignoring the variance heterogeneity. Given the variance components the mean function is estimated by smoothing splines as the minimizer of the penalized weighted least squares. Given the mean function, we propose a likelihood ratio test statistic for identifying the variance change point. The null distribution of the test statistic is derived together with the rates of convergence of all the parameter estimates. Simulations show excellent performance of the proposed method. Application analysis offers numerical support to non-invasive organ viability assessment by surface temperature monitoring. Extension to functional variance change point detection will also be presented if time allows.
Spring 2020
Spring 2020 Colloquia will take place Mondays, 3:30pm in Math 501, unless otherwise noted.
- February 3, 2020 - Speaker - Ian McKeague, Department of Biostatistics, Columbia University
Title: Functional data analysis for activity profiles from wearable devices
Abstract: This talk introduces a nonparametric framework for analyzing physiological sensor data collected from wearable devices. The idea is to apply the stochastic process notion of occupation times to construct activity profiles that can be treated as monotonically decreasing functional data.
Whereas raw sensor data typicallyAneed to be pre-aligned before standard functional data methods are applicable, activity profiles are automatically aligned because they are indexed by activity level rather than by follow-up time. We introduce a nonparametric likelihood ratio approach that makes efficient use of the activity profiles to provide a simultaneous confidence band for their mean (as a function of activity level), along with an ANOVA type test. These procedures are calibrated using bootstrap resampling. Unlike many nonparametric functional data methods, smoothing techniques are not needed. Accelerometer data from subjects in a U.S. National Health and Nutrition Examination Survey (NHANES) are used to illustrate the approach. The talk is based on joint work with Hsin-wen Chang (Academia Sinica, Taipei).
- March 2, 2020 - Speaker - Yichuan Zhao, Department of Professor Mathematics and Statistics, Georgia State University
Title: Penalized Empirical Likelihood for the Sparse Cox Model
Abstract: The current penalized regression methods for selecting predictor variables and estimating the associated regression coefficients in the Cox model are mainly based on partial likelihood. In this paper, an empirical likelihood method is proposed for the Cox model in conjunction with appropriate penalty functions when the dimensionality of data is high. Theoretical properties of the resulting estimator for the large sample are proved. Simulation studies suggest that empirical likelihood works better than partial likelihood in terms of selecting correct predictors without introducing more model errors. The well-known primary biliary cirrhosis data set is used to illustrate the proposed empirical likelihood method.
This is joint work with Dongliang Wang and Tong Tong Wu.
Fall 2019
Fall 2019 colloquia will take place Mondays, 3:30 to 4:30pm in MATH 501, unless otherwise noted.
- September 9, 2019 - Speaker - Hui Zou, Department of Statistics, University of Minnesota
Title: A nearly condition-free fast algorithm for Gaussian graphical model recovery
Abstract: Many methods have been proposed for estimating Gaussian graphical model. The most popular ones are the graphical lasso and neighborhood selection, because the two are computational very efficient and have some theoretical guarantees. However, their theory for graph recovery requires some very stringent structure assumption (a.k.a. the irrepresentable condition). We argue that replacing the lasso penalty in these two methods with a non-convex penalty does not fundamentally remove the theoretical limitation, because another structure condition is required. As an alternative, we propose a new algorithm for graph recovery that is very fast and easy to implement and enjoys strong theoretical properties under basic sparsity assumptions.
- October 14, 2019 - Speaker - Jian Liu, Associate Professor, Systems and Industrial Engineering, University of Arizona
Title: Functional Data Analytics for Detecting Bursts in Water Distribution Systems
Abstract: Bursts in water distribution systems (WDSs) are a special type of short-term, high-flow water loss that can be a significant component of a system’s water balance. Since WDSs are usually deployed underground, bursts are difficult to be detected before their catastrophic results are observed on the ground surface. Continuous hydraulic data streams collected from automatic meter reading and advanced metering infrastructure systems make it possible to detect bursts in WDS based on data analytics. Existing methods based on conventional statistical process control charts may not be effective, as the temporal correlations imbedded in the data streams are not explicitly considered. In this seminar, new control charts for burst detection based on functional data analysis will be presented. Both Phase-I and Phase-II monitoring schemes are investigated. The temporal correlations are modeled from empirical data streams continuously collected from the same WDS. Their statistical properties are studied to reflect system inherent uncertainties induced by customers’ daily use without bursts. The bursts are detected by comparing the new hydraulic data stream to the inherent uncertainties through statistical control charting. The new method will significantly reduce the rate of false alarm and miss detection. The effectiveness of the proposed method is demonstrated with a case study based on numerical simulation of a real-world WDS.
- November 12, 2019 - Speaker - Dennis Lin, Department of Statistics, Penn State University **NOTE** Tuesday at 1:00pm in Math 501
Title: Interval Data: Modeling and Visualization
Abstract: Interval-valued data is a special symbolic data composed of lower and upper bounds of intervals. It can be generated from the change of climate, fluctuation of stock prices, daily blood pressures, aggregation of large datasets, and many other situations. Such type of data contains rich information useful for decision making. The prediction of interval-valued data is a challenging task as the predicted lower bounds of intervals should not cross over the corresponding upper bounds. In this project, a regularized artificial neural network (RANN) is proposed to address this difficult problem. It provides a flexible trade-off between prediction accuracy and interval crossing. Empirical study indicates the usefulness and accuracy of the proposed method. The second portion of this project provides some new insights for visualization of interval data. Two plots are proposed—segment plot and dandelion plot. The new approach compensates the existing visualization methods and provides much more information. Theorems have been established for reading these new plots. Examples are given for illustration.
- December 2, 2019 - Speaker - Wenguang Sun, Associate Professor, Dept of Data Sciences and Operations, USC
Title: Large-Scale Estimation and Testing Under Heteroscedasticity
Abstract: The simultaneous inference of many parameters, based on a corresponding set of observations, is a key research problem that has received much attention in the high-dimensional setting. Many practical situations involve heterogeneous data where the most common setting involves unknown effect sizes observed with heteroscedastic errors. Effectively pooling information across samples while correctly accounting for heterogeneity presents a significant challenge in large-scale inference. The first part of my talk addresses the selection bias issue in large-scale estimation problem by introducing the “Nonparametric Empirical Bayes Smoothing Tweedie” (NEST) estimator, which efficiently estimates the unknown effect sizes and properly adjusts for heterogeneity via a generalized version of Tweedie’s formula. The second part of my talk focuses on a parallel issue in multiple testing. We show that there can be a significant loss in information from basing hypothesis tests on standardized statistics rather than the full data. We develop a new class of heteroscedasticity–adjusted ranking and thresholding (HART) rules that aim to improve existing methods by simultaneously exploiting commonalities and adjusting heterogeneities among the study units. The common message in both NEST and HART is that the variance structure, which is subsumed under standardized statistics, is highly informative and can be exploited to achieve higher power in both shrinkage estimation and multiple testing problems.
- December 9, 2019 - Speaker - Vladimir Minin, Department of Statistics, UC Irvine
Title: Statistical challenges and opportunities in stochastic epidemic modeling
Abstract: Stochastic epidemic models describe how infectious diseases spread through populations. These models are constructed by first assigning individuals to compartments (e.g., susceptible, infectious, and removed) and then defining a stochastic process that governs the evolution of sizes of these compartments through time. Stochastic epidemic models and their deterministic counterparts are useful for evaluating strategies for controlling the infectious disease spread and for predicting the future course of a given epidemic. However, fitting these models to data turns out to be a challenging task, because even the most vigilant infectious disease surveillance programs offer only noisy snapshots of the number of infected individuals in the population. Such indirect observations of the infectious disease spread result in high dimensional missing data (e.g., number and times of infections) that needs to be accounted for during statistical inference. I will demonstrate that combining stochastic process approximation techniques with high dimensional Markov chain Monte Carlo algorithms makes Bayesian data augmentation for stochastic epidemic models computationally tractable. I will present examples of fitting stochastic epidemic models to incidence time series data collected during outbreaks of Influenza and Ebola viruses.